Forex & Crypto
From Novice to Expert: How Forex Robots Can Fast-Track Your Trading Journey
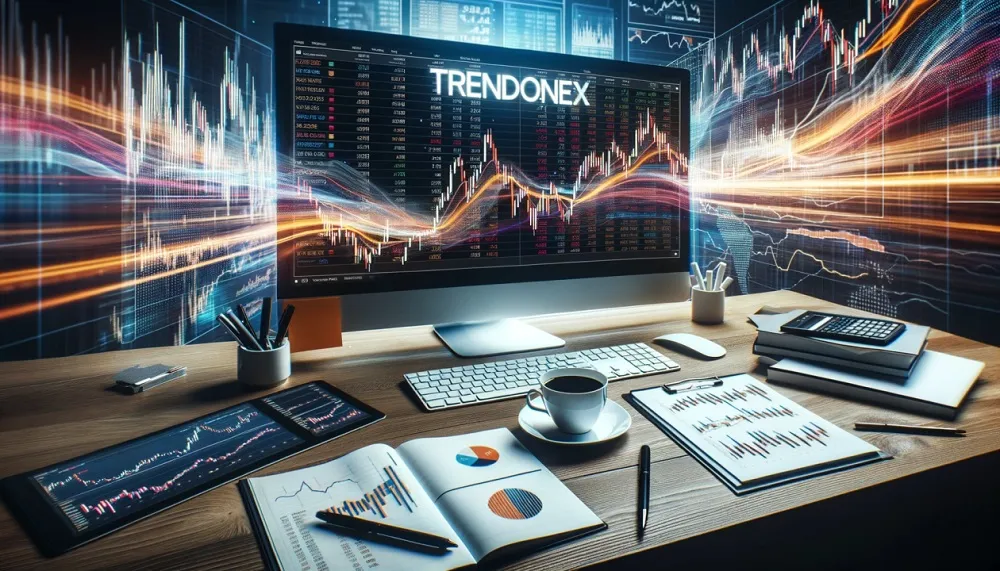
Embarking on a journey into the world of Forex trading can be both exhilarating and daunting for novices. With its potential for substantial profits and simultaneous risks, navigating the Forex market requires a blend of skill, strategy, and experience. However, for those looking to accelerate their learning curve and fast-track their trading journey, the emergence of Forex robot offers a promising solution. This article explores how Forex robots can expedite the transition from novice to expert in Forex trading, empowering traders to achieve success more rapidly and confidently.
The Beginner’s Challenge
For novice traders, the Forex market can be a labyrinth of complexities and uncertainties. Understanding market dynamics, mastering technical analysis, and developing effective trading strategies are just a few of the challenges that beginners must overcome. Additionally, navigating the emotional rollercoaster of trading, managing risk, and maintaining discipline can further complicate the learning process. As a result, many novice traders struggle to achieve consistent profitability and may experience setbacks along their trading journey.
The Role of Forex Robots
Forex robots, also known as expert advisors (EAs), are automated trading systems designed to execute trades in the Forex market based on predefined rules and algorithms. These algorithms are crafted using advanced mathematical models, technical indicators, and trading strategies, enabling the robot to analyze market data and identify trading opportunities with precision and efficiency. By automating the trading process, Forex robots help novice traders overcome the challenges of trading and fast-track their journey to success.
Accelerated Learning Curve
One of the key advantages of using Forex robots is their ability to expedite the learning curve for novice traders. Instead of spending years mastering complex trading strategies and techniques, novice traders can leverage Forex robots to execute trades on their behalf. This allows them to observe the performance of various trading strategies in real-time, gain insights into market dynamics, and learn from the outcomes of their trades. By providing hands-on experience and real-time feedback, Forex robots enable novice traders to accelerate their learning process and gain valuable insights into the intricacies of Forex trading.
Emotion-Free Trading
Emotions such as fear, greed, and impatience can often cloud the judgment of novice traders, leading to irrational decision-making and suboptimal outcomes. Forex robots operate based on predefined rules and algorithms, free from emotional biases. By eliminating emotional influences from the trading process, Forex robots enable novice traders to maintain discipline and consistency in their trading strategies, leading to more predictable and profitable results. This allows novice traders to focus on learning and improving their trading skills without being hindered by emotional distractions.
Risk Management
Effective risk management is crucial for novice traders to protect their capital and minimize losses. Forex robots can be programmed to implement risk management measures such as stop-loss orders and position sizing rules to limit losses and protect capital. Additionally, Forex robots can diversify trading across multiple currency pairs and markets to spread risk and reduce exposure to any single trade or asset. By providing robust risk management capabilities, Forex robots help novice traders navigate the Forex market more safely and confidently, mitigating the risks associated with trading.
Backtesting and Optimization
Before deploying a trading strategy in live market conditions, novice traders can use Forex robots to backtest and optimize their strategies. Backtesting involves simulating the performance of a trading strategy over historical market data to assess its viability and profitability. By conducting rigorous backtesting, novice traders can identify potential weaknesses in their strategies and make necessary adjustments to optimize performance and maximize returns. This enables novice traders to gain confidence in their trading strategies and make informed decisions when trading in live market conditions.
Conclusion
In conclusion, Forex robots offer a valuable tool for novice traders looking to fast-track their trading journey and achieve success in the Forex market. By automating the trading process, providing hands-on experience, and offering valuable insights into market dynamics, Forex robots enable novice traders to accelerate their learning curve and gain confidence in their trading abilities. Additionally, Forex robots provide robust risk management capabilities and facilitate backtesting and optimization, further enhancing the success potential of novice traders. As technology continues to advance, the role of Forex robots in empowering novice traders is expected to expand further, unlocking new opportunities for success in the dynamic world of Forex trading.
Forex & Crypto
Enhancing Risk Management with Dynamic Position Sizing in Forex Robot Strategies
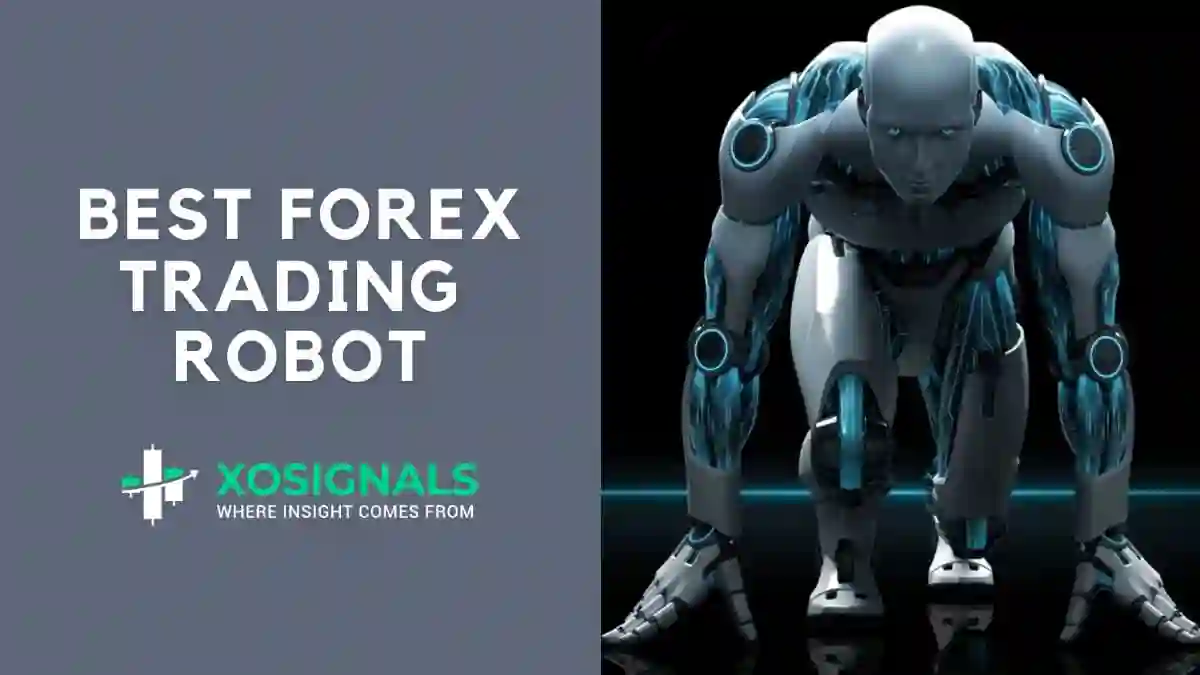
In the realm of Forex trading, where market volatility and uncertainty abound, effective risk management is paramount to long-term success. Forex robots, automated trading systems driven by predefined algorithms, offer traders a means to execute trades with precision and consistency. However, to mitigate the inherent risks associated with Forex trading, incorporating dynamic position sizing strategies into Forex robot strategies is essential. This article explores the significance of dynamic position sizing in Forex trading, its benefits, applications, and techniques for implementation to enhance risk management.
Understanding Dynamic Position Sizing in Forex Trading
Position sizing refers to the allocation of capital to individual trades based on predetermined risk parameters, such as the percentage of account equity at risk per trade or the distance to the stop-loss level. Dynamic position sizing involves adjusting the size of each trade dynamically based on changing market conditions, account equity, and risk appetite. By dynamically resizing positions, traders can optimize risk-reward ratios, maximize returns, and minimize the impact of drawdowns on their trading capital.
The Importance of Dynamic Position Sizing in Forex Robot Strategies
- Optimizing Risk-Reward Ratios: Dynamic position sizing allows traders to adjust trade sizes based on the perceived risk of each trade relative to its potential reward. By allocating more capital to high-probability trades with favorable risk-reward ratios and scaling back on lower-probability trades, traders can optimize their overall risk-adjusted returns.
- Adapting to Changing Market Conditions: Forex markets are dynamic and prone to fluctuations in volatility, liquidity, and trend strength. Dynamic position sizing enables traders to adapt their trade sizes to changing market conditions, scaling up during periods of high volatility and scaling down during periods of low volatility to maintain consistent risk exposure.
- Managing Account Drawdowns: By limiting the size of each trade relative to account equity and risk tolerance, dynamic position sizing helps traders manage drawdowns and preserve capital during losing streaks. By reducing position sizes during drawdowns and increasing sizes during winning streaks, traders can smooth out equity curves and mitigate the impact of losses on overall portfolio performance.
- Enhancing Consistency: Dynamic position sizing promotes consistency in trading performance by ensuring that each trade is sized appropriately based on risk parameters and market conditions. By maintaining consistent risk exposure across trades, traders can achieve more predictable and stable returns over time.
Techniques for Implementing Dynamic Position Sizing in Forex Robot Strategies
- Percentage Risk Model: The percentage risk model allocates a fixed percentage of account equity to each trade, based on predefined risk parameters such as maximum allowable drawdown or percentage of account equity at risk per trade. This model ensures consistent risk exposure across trades while adapting trade sizes to changes in account equity.
- Volatility-based Position Sizing: Volatility-based position sizing adjusts trade sizes dynamically based on the volatility of the underlying currency pair. Higher volatility pairs may warrant smaller trade sizes to limit potential losses, while lower volatility pairs may allow for larger trade sizes to capture potential gains.
- ATR (Average True Range) Method: The ATR method calculates trade sizes based on the average true range of the currency pair, a measure of volatility. Trades are sized proportionally to the ATR value, with larger ATR values corresponding to smaller trade sizes and vice versa. This method ensures that trade sizes are aligned with current market volatility levels.
- Monte Carlo Simulation: Monte Carlo simulation involves simulating multiple scenarios of market outcomes based on historical data and varying trade sizes to assess the impact on account equity and drawdowns. By running Monte Carlo simulations, traders can identify optimal trade sizes that balance risk and reward and maximize long-term profitability.
Benefits of Dynamic Position Sizing in Forex Robot Strategies
- Improved Risk Management: Dynamic position sizing enables traders to manage risk more effectively by adjusting trade sizes in response to changing market conditions and account equity levels. By limiting risk exposure and preserving capital during drawdowns, traders can achieve more consistent and sustainable returns over time.
- Enhanced Performance: By optimizing trade sizes based on risk parameters and market conditions, dynamic position sizing maximizes risk-adjusted returns and enhances overall trading performance. Traders can capitalize on favorable opportunities while minimizing the impact of adverse market movements on portfolio performance.
- Adaptability: Dynamic position sizing strategies are adaptable to different trading styles, time frames, and market conditions. Whether trading short-term trends or long-term reversals, dynamic position sizing allows traders to tailor their risk management approach to suit their specific trading objectives and preferences.
- Reduced Emotional Bias: By automating position sizing decisions based on predefined rules and parameters, dynamic position sizing eliminates emotional bias from trading decisions. Traders can execute trades with discipline and consistency, free from the influence of fear, greed, or other emotions that may cloud judgment.
Conclusion
Dynamic position sizing is a critical component of effective risk management in Forex trading, particularly when employing Forex robot strategies. By adjusting trade sizes dynamically based on changing market conditions, account equity, and risk parameters, traders can optimize risk-reward ratios, manage drawdowns, and enhance overall trading performance. Whether using percentage risk models, volatility-based sizing, ATR methods, or Monte Carlo simulations, dynamic position sizing offers a versatile and adaptable approach to risk management that can be tailored to suit individual trading styles and objectives. By incorporating dynamic position sizing into Forex robot strategies, traders can achieve more consistent and sustainable returns while mitigating the inherent risks of Forex trading.
Forex & Crypto
Enhancing Adaptability with Reinforcement Learning in Forex Robot Strategies
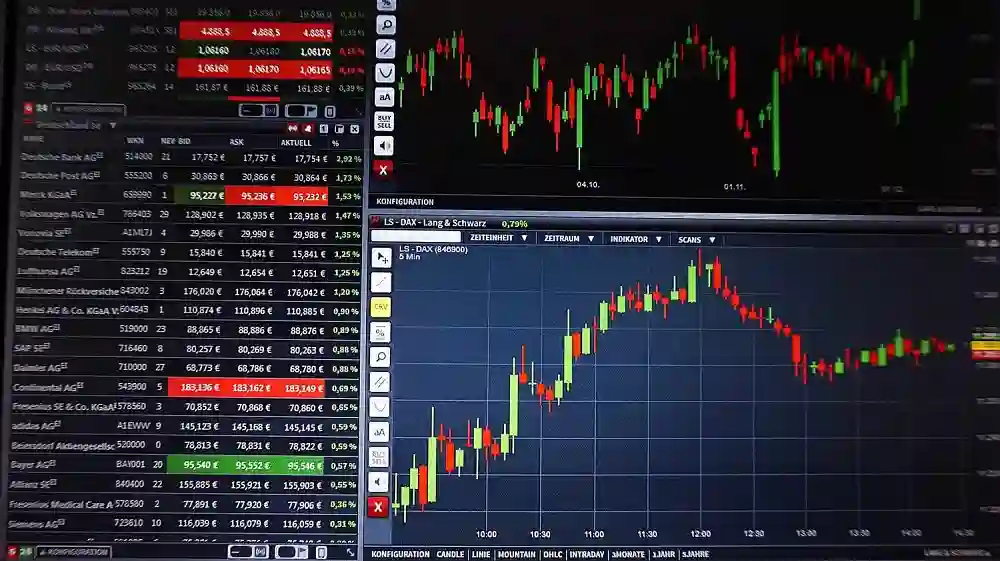
In the fast-paced and ever-changing world of forex trading, adaptability is key to success. Market conditions can shift rapidly, presenting new challenges and opportunities for traders. To thrive in this dynamic environment, forex robot strategies must be capable of adapting to changing market dynamics effectively. One approach to achieving this adaptability is through reinforcement learning (RL), a branch of machine learning that enables agents to learn optimal actions through trial and error interactions with the environment. In this article, we explore how reinforcement learning can enhance adaptability in forex robot strategies, its benefits, challenges, and implications for trading performance.
Understanding Reinforcement Learning in Forex Trading
Reinforcement learning is a machine learning paradigm inspired by behavioral psychology, where an agent learns to make decisions by interacting with an environment to maximize cumulative rewards. In the context of forex trading, the environment comprises historical market data, price movements, technical indicators, and economic variables, while the agent is the forex robot tasked with making trading decisions.
The RL agent learns to navigate the forex market by taking actions, observing outcomes, and receiving feedback in the form of rewards or penalties. Over time, the agent learns optimal trading strategies that maximize rewards and adapt to changing market conditions.
Benefits of Reinforcement Learning in Forex Trading
Reinforcement learning offers several benefits for forex trading:
- Adaptability: RL agents can adapt to changing market conditions and learn optimal trading strategies through trial and error interactions with the environment. Unlike rule-based strategies that rely on predefined rules, RL agents continuously learn from experience and adjust their behavior accordingly.
- Complex Decision-Making: RL agents can handle complex decision-making tasks in the forex market, such as portfolio optimization, risk management, and position sizing. By learning from past experiences and feedback, RL agents can develop sophisticated trading strategies that maximize returns while minimizing risk.
- Dynamic Optimization: RL agents can dynamically optimize trading strategies based on real-time market data and feedback. They can adjust parameters, entry/exit criteria, and risk management rules in response to changing market dynamics, ensuring adaptability and robustness in various market conditions.
- Exploration and Exploitation: RL agents balance exploration (trying new actions to discover optimal strategies) and exploitation (leveraging known strategies to maximize rewards) to achieve a balance between learning and performance. This enables RL agents to discover new trading opportunities while leveraging proven strategies for consistent returns.
- Learning from Uncertainty: RL agents can learn from uncertainty and volatility in the forex market, adapting their strategies to minimize losses during periods of market turbulence and capitalize on opportunities during periods of stability.
Implementation Strategies for Reinforcement Learning in Forex Robot Strategies
Traders can implement reinforcement learning in forex robot strategies using the following strategies:
- State Representation: Define the state space of the RL agent, comprising relevant market variables, technical indicators, and economic factors. Choose appropriate state representations that capture key features of the forex market and enable the agent to make informed trading decisions.
- Action Space: Define the action space of the RL agent, comprising possible trading actions, such as buy, sell, hold, or adjust position sizes. Design a discrete or continuous action space that allows the agent to explore different trading strategies and optimize performance.
- Reward Function: Design a reward function that provides feedback to the RL agent based on trading outcomes. Define rewards to incentivize profitable trades, penalize losses, and encourage behaviors that align with trading objectives, such as risk-adjusted returns or drawdown minimization.
- Training Environment: Create a simulated trading environment or use historical market data to train the RL agent. Implement a trading simulator that mimics real-world trading conditions, allowing the agent to learn from past experiences and interactions with the environment.
- Algorithm Selection: Choose appropriate RL algorithms, such as Q-learning, Deep Q-Networks (DQN), Policy Gradient methods, or Actor-Critic algorithms, based on the complexity of the trading task and the size of the state and action spaces. Experiment with different algorithms to find the most suitable approach for your forex robot strategy.
Challenges and Considerations
While reinforcement learning offers significant potential for enhancing adaptability in forex robot strategies, traders should be aware of potential challenges and considerations:
- Sample Efficiency: RL algorithms may require large amounts of training data and computational resources to learn optimal trading strategies effectively. Traders should consider strategies to improve sample efficiency, such as data augmentation, transfer learning, or model pretraining.
- Overfitting: RL agents may overfit to past market data, resulting in poor generalization performance and suboptimal trading strategies. Traders should use techniques such as regularization, dropout, or ensemble methods to mitigate overfitting risks and ensure robustness in live trading environments.
- Hyperparameter Tuning: RL algorithms contain hyperparameters that must be tuned to optimize performance. Traders should experiment with different hyperparameter settings using techniques such as grid search, random search, or Bayesian optimization to find the optimal configuration for their forex robot strategy.
- Model Interpretability: RL agents may be black-box systems, making it challenging to interpret their decisions and understand the reasoning behind trading strategies. Traders should strive to improve model interpretability using techniques such as attention mechanisms, saliency maps, or model visualization tools.
- Real-time Execution: Real-time execution of RL-based forex robot strategies requires low-latency trading infrastructure and efficient implementation to respond to changing market conditions quickly. Traders should optimize code performance, minimize latency, and implement efficient order execution algorithms to ensure timely trade execution.
Conclusion
Reinforcement learning offers a powerful framework for enhancing adaptability in forex robot strategies, enabling agents to learn optimal trading strategies through trial and error interactions with the environment. By leveraging RL algorithms, traders can develop forex robot strategies that adapt dynamically to changing market conditions, optimize performance, and capitalize on emerging opportunities. While challenges exist, including sample efficiency, overfitting risks, hyperparameter tuning, and model interpretability, the potential benefits of reinforcement learning in forex trading justify its adoption as a fundamental tool for achieving trading success in today’s dynamic and unpredictable markets. As technology continues to evolve and RL algorithms become more sophisticated, the integration of reinforcement learning is likely to play an increasingly prominent role in shaping the future of forex robot strategies and algorithmic trading.
Forex & Crypto
The Impact of Artificial Intelligence on Forex Robot Trading
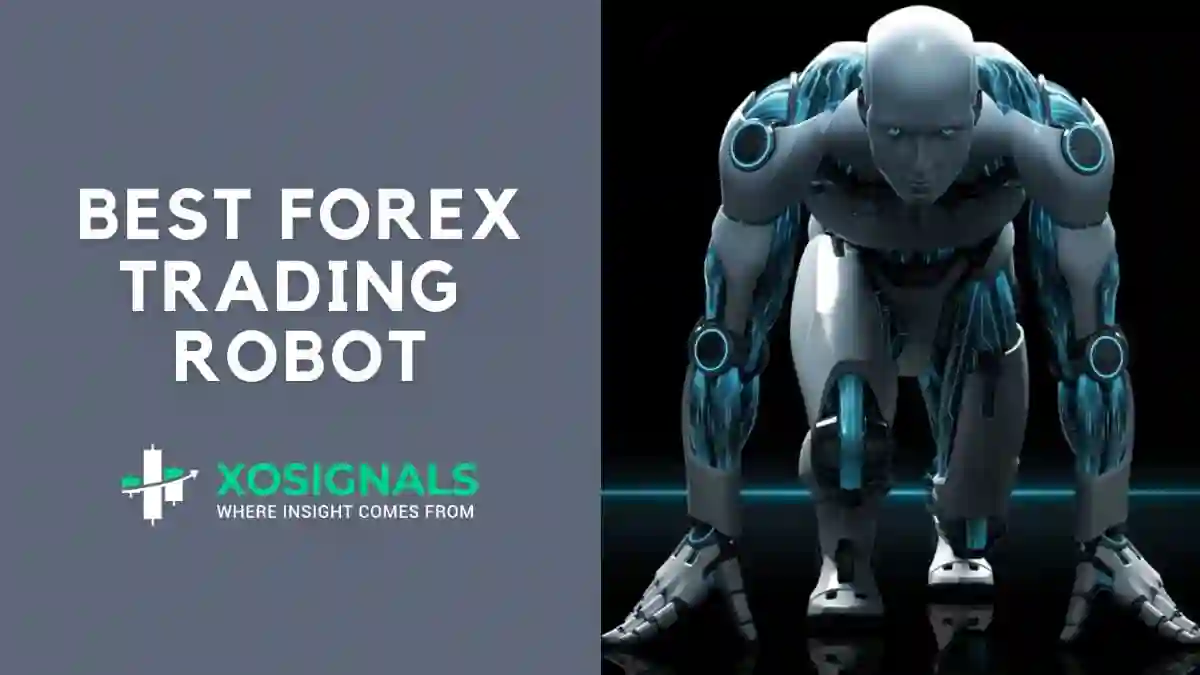
Artificial intelligence (AI) has revolutionized the landscape of forex robot trading, ushering in a new era of advanced algorithms, adaptive strategies, and enhanced decision-making capabilities. This article explores the profound impact of AI on forex robot trading, delving into key advancements, innovative applications, and the implications for traders seeking to capitalize on the dynamic forex market.
The fusion of artificial intelligence and forex robot trading has reshaped the way traders engage with the forex market, empowering them with intelligent tools capable of analyzing vast amounts of data, identifying patterns, and executing trades with precision. AI-driven forex robots leverage machine learning algorithms, neural networks, and natural language processing to adapt to changing market conditions, optimize trading strategies, and enhance performance. This article illuminates the transformative impact of AI on forex robot trading, uncovering the myriad ways in which advanced algorithms are shaping the future of currency trading.
The Evolution of AI in Forex Robot Trading:
From Rule-Based Systems to Adaptive Algorithms:
Early forex robots relied on rule-based systems and simple algorithms to execute trades based on predefined criteria. However, the advent of AI revolutionized forex robot trading by introducing adaptive algorithms capable of learning from data and adjusting strategies in real-time. Machine learning techniques, such as supervised learning, unsupervised learning, and reinforcement learning, enable robots to analyze market dynamics, identify patterns, and adapt trading strategies to evolving conditions.
Predictive Analytics and Pattern Recognition:
AI-powered forex robots excel at predictive analytics and pattern recognition, leveraging historical data and real-time market feeds to forecast price movements and identify trading opportunities. By analyzing vast datasets and detecting subtle patterns that may elude human traders, AI-driven robots can anticipate market trends, predict price reversals, and optimize entry and exit points for trades.
Sentiment Analysis and News-Based Trading:
Natural language processing (NLP) algorithms enable forex robots to analyze news sentiment, social media feeds, and economic indicators to gauge market sentiment and assess the impact of news events on currency prices. Sentiment analysis helps robots make informed trading decisions based on market sentiment, news sentiment, and macroeconomic factors, thereby enhancing risk management and strategy optimization.
High-Frequency Trading and Low-Latency Execution:
AI-powered forex robots are well-suited for high-frequency trading (HFT) strategies, leveraging ultra-fast execution speeds and low-latency connectivity to capitalize on fleeting market inefficiencies and exploit price discrepancies. By leveraging advanced algorithms and low-latency infrastructure, AI-driven robots can execute trades with millisecond precision, enabling traders to profit from rapid market movements and arbitrage opportunities.
Innovative Applications of AI in Forex Robot Trading:
Adaptive Learning and Optimization:
AI-driven forex robots employ adaptive learning techniques to continuously refine trading strategies and adapt to changing market conditions. Through iterative learning and optimization, robots can identify and exploit evolving market trends, adjust risk parameters dynamically, and optimize performance over time. Adaptive algorithms enable robots to evolve and improve their trading capabilities, leading to enhanced profitability and resilience.
Portfolio Diversification and Risk Management:
AI-powered forex robots facilitate portfolio diversification and risk management by optimizing asset allocation, adjusting position sizes, and dynamically hedging risk exposure. By analyzing correlations, volatility, and covariance matrices, robots construct diversified portfolios that balance risk and return across multiple currency pairs, asset classes, and trading strategies. Advanced risk management features such as stop-loss orders, take-profit targets, and dynamic position sizing help mitigate risk and preserve capital in volatile market conditions.
Deep Learning and Neural Networks:
Deep learning algorithms and neural networks empower forex robots with advanced pattern recognition capabilities, enabling them to identify complex patterns and trends in market data. By leveraging hierarchical architectures and multiple layers of abstraction, deep learning models can extract valuable insights from unstructured data, such as price charts, technical indicators, and news articles. Neural networks enable robots to make informed trading decisions based on learned patterns and correlations, leading to more accurate predictions and improved trading performance.
The Implications for Traders:
Enhanced Trading Efficiency and Performance:
AI-driven forex robots offer traders enhanced trading efficiency and performance by leveraging advanced algorithms and predictive analytics to optimize trading strategies and capitalize on market opportunities. By automating decision-making processes and adapting to changing market conditions, AI-powered robots enable traders to achieve better results with less effort and time investment.
Improved Risk Management and Capital Preservation:
AI-powered forex robots enhance risk management and capital preservation by employing sophisticated algorithms and dynamic risk parameters to mitigate downside risk and protect trading capital. By diversifying portfolios, adjusting position sizes, and implementing risk controls, robots help traders manage risk exposure and minimize losses in volatile market conditions.
Access to Advanced Tools and Insights:
AI-driven forex robots provide traders with access to advanced tools and insights, such as predictive analytics, sentiment analysis, and pattern recognition, that enable them to make more informed trading decisions and optimize performance. By leveraging AI technology, traders can gain valuable insights into market trends, identify profitable opportunities, and execute trades with greater precision and confidence.
Conclusion:
The integration of artificial intelligence into forex robot trading has revolutionized the way traders engage with the forex market, offering advanced algorithms, adaptive strategies, and enhanced decision-making capabilities. AI-driven forex robots leverage machine learning, natural language processing, and deep learning techniques to analyze market data, predict price movements, and optimize trading strategies in real-time. By harnessing the power of AI, traders can enhance trading efficiency, improve risk management, and capitalize on market opportunities with greater precision and confidence, shaping the future of currency trading in the digital age.
-
Social Media8 months ago
6 Things You Need to Know About Buying YouTube Comments
-
Safety & Security10 months ago
How can education helps in attaining safe and security?
-
Education11 months ago
Literature Gap: What It Means And How To Find It
-
Technology10 months ago
15 Different Types of Technology We Use Everyday
-
Education4 months ago
Overcoming Challenges in Online Degree Programs: Tips for Students
-
Marketing10 months ago
Top 12 Marketing Agencies to Grow Your Business in 2023
-
Business10 months ago
How to Write a Startup Business Plan
-
Forex & Crypto10 months ago
Top 7 Forex Trading Strategies For Beginners